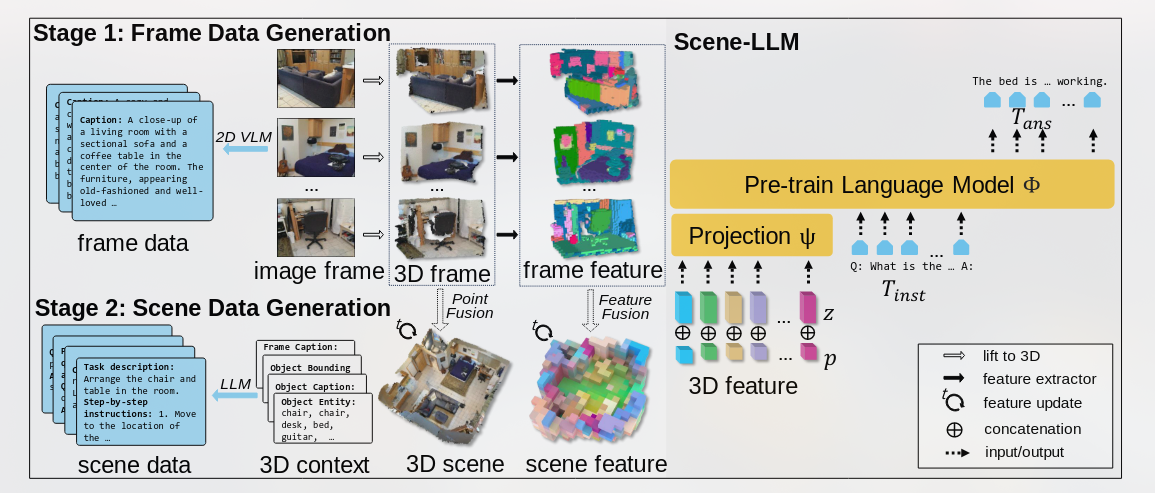
https://github.com/IDEA-Research/Grounded-Segment-Anything
By [[Grounding-DINO]] + SAM
Achieving Open-Vocab. Det & Seg
https://github.com/IDEA-Research/Grounded-Segment-Anything
By [[Grounding-DINO]] + SAM
Achieving Open-Vocab. Det & Seg
本文提出的模型主要想解决3D密集标注和交互式规划。
结合
需要align the dense 3D visual information with the textual embedding space of a pre-trained LLM。3D点集由于其连续坐标系以及需要适应场景状态变化的表示形式而构成了一个独特的问题
3D-VQA
VLN(Visual-Language Navigation)
和[[3D-LLM]]一样,都是多视角采集D-RGB信息然后整合为3D frame
标注信息来自于Mini-GPT-V2(capable of generating captions and object descriptions from images by using caption and grounded caption identifiers)。
Uses image frames and a 2D-VLM(Mini-GPT-V2) to generate frame descriptions
3D场景数据是通过基于其相机姿势汇总的3D帧来重建
使用Llama-2-Chat-70B [65]生成场景的语言注释
From Vision Studio 对于VLM生成内容使用的self-checking: [83]prompted with a mix of context data including generated frame captions, frame object descriptions, annotated object lists, and annotated bounding boxes. These prompts lead to diverse instruction-following data types like dense caption, object caption, task decomposition, functionality enhancement, question-answering, and human-robot dialogues
场景-LLM是一种3D视觉语言模型(VLM),具有简单而有效的体系结构,旨在理解以基于本体和场景级别的3D视觉信息,使其能够成功执行交互式计划任务。本节概述了3D视觉特征提取过程,我们的模型的体系结构,3D视觉信息与数据集的对齐以及使用Scene-LLM进行推理。
Employ visual language semantic features [51] to represent 3D visual semantics
Tokenize 3D visual features for LLM input:
To bridge 3D visual tokens(F) with the LLM’s tokenized space
FC(1030, 768)->GELU->FC(768,768)
Llama-2-7b as the foundational LLM backbone
在两个坐标系统(camera和世界坐标)下使用3D帧数据,以确保场景-LLM理解以自我为中心和以场景为中心的观点。
在此阶段,仅训练了projection layer,可以有效地对齐具有文本特征的3D视觉特征,同时保持LLM参数(φ)不变。
优化Scene-llm,以准确响应用户说明。我们使用标识符令牌“我看到”将3D帧语言和3D场景语言数据合并到前言。文本描述分为指令($T_{INST}$)及其相应的响应($T_{ANS}$)。利用转换后的3D视觉令牌($T_{3D}$)和指令令牌($T_{INST}$),我们的目标是微调LLM(φ)以自动生成$T_{ANS}$.
在这里,我们共同微调了投影层和LLM,由θ= {ψ,φ}表示
在读这本书之前从各种渠道了解了很多推荐语
得承认在此之前我对于印度的认知仅限于《三傻大闹宝莱坞》和历史课本中对于其历史文化的只言片语(以及我根本不求甚解)。书中的印度是否真实我并不关心,但我确实感受到了“黑塞心中禅宗的国度会是什么样的“。在这里,最为尊贵每天的日程便是,沐浴,诵经,聆听佛陀;在这里人们为了拜见一位在世活佛,甘愿跋涉千里,抛弃一切,只求得听其法义,以洗涤心灵;在这里,一个人的虔诚,洁净,可以令金钱黯然失色。
西游记中西天取的经,是无字经书,大道无言这一点在书中也有体现。
知识可以传授,但智慧不能。人们可以寻见智慧,在生命中体现出智慧,以智慧自强,以智慧来创造奇迹,但人们不可能去传授智慧。我年少时就有过这种疑问,正是我的怀疑驱使我远离教师们。我还有过一种思想,乔文达,你又会认为那是开玩笑或只是一种愚蠢的念头:就是说,每一真理的反面也同样真实。比如说,只有偏面的真理才能形诸于言辞;事实上,以语言表达或思维的一切都只能是片面的,只是半个真理而已,它们都缺乏完备、圆融与统一;当佛陀世尊宣讲关于世界的教义,他不得不把世界分为轮回与涅槃,虚幻与真如,痛苦与救赎。人别无选择,对于那些要传授教义的导师们来说尤其如此。而世界自身则遍于我之内外,从不片面。从未有一人或一事纯属轮回或者纯属涅槃,从未有一人完全是圣贤或是罪人。世界之所以表面如此是因为我们有一种幻觉,即认为时间是某种真实之物。时间并无实体,乔文达,我曾反复悟到这一点。而如果时间并非真实,那么仿佛存在于现世与永恒,痛苦与极乐,善与恶之间的分界线也只是一种幻象。
悉达多在自我的路上深沉地思索。他意识到自己已不再是青年,他已经是一个成熟的男人。他感到某种东西已然脱离了他,仿佛一条蛇已蜕去了旧皮。那种伴随他整个青年时代并一直是他自我之一部分的因素已被抛在了身后:这就是寻觅导师和聆听教义的愿望。他已经离开他所见到过的最后一位导师——这位贤明的导师,最神圣的佛陀世尊。他甚至不得不离开他,他无法接受他的教义。
这位思想者缓缓地走自己的路并向自己道:你想要从教义和教师那里学到,而尽管他们教给你许多,却无法传授与你的那件事到底是什么呢? 他接着想:那就是自我,我希望学到有关自我的特性与本质。过去我一直想要摆脱自我并征服自我,然而我从未能够征服自我,我只是在欺骗它,逃离它,躲避它。的确,世上没有任何东西能够如自我那样占据我全部的思绪。这是一个难解之谜:我存在,而且我是唯一的、不同于任何其他人的独立个体。我是悉达多。我对世上万有所知最少的恰恰是我的自我,恰恰是悉达多。
这位踽踽独行的沉思者突然停下来,为那种意念所攫住,而另一种意念会立刻从前念之中浮现出来,这就是:之所以我对自我一无所知,之所以悉达多对我来说一直保持陌生与未知,只因为一点,只由于这唯一的原因——我害怕自我,我在逃避自我。我在追寻梵天,阿特曼。我欲求摧毁自我、摆脱自我以便在未知的存在最深层发现万有的核心,即阿特曼、生命、上帝或绝对终极之物。而正因为如此,我却一路丢失了自我。
他举目四望,仿佛初次见到这个世界。这世界美丽、陌生而神秘。瞧! 那儿是金黄,那儿是湛蓝,那儿又是碧绿。天空与河流,森林和山峦都如此美好,如此神秘,如此诱人,而在所有这一切之中,他,觉醒的悉达多,正走在通向自我的道路上。所有这一切,所有这些金黄与湛蓝,河流与树木第一次映入悉达多的眼帘。那不再是魔罗魅惑迷人的幻术,不再是玛耶虚无缥缈的面纱,也不再是世界万像毫无意义的偶然显现。尽管这一切为那些追求圆融统一,轻视个别差异的思想高深的婆罗门贵族所鄙弃,而河流毕竟就是河流。假如悉达多自性中唯一的神明隐秘地居于湛蓝或河流之中,那么此刻恰恰是金黄与湛蓝、天空与森林映入站在这里的悉达多的眼帘,这本身也正是天意,正是神的安排。意义与实在并非隐藏于事物的背后,而是寓于事物自身,寓于事物的一切现象。
尘世并非不圆满,或是正处在一条通向圆满的漫长的路上。不,他每一个瞬间都是圆满的。一切罪孽本身就蕴含着宽恕,所有小孩儿本身就蕴含着老人,所有新生儿都蕴含着死亡,所有濒死者都蕴含着永生。
他看到的不再是他的朋友悉达多的脸庞,他仿佛看到许许多其他的形象,一个长长的系列,一条不息的形象之流——百种,千种,万种,无数的形象不断生生灭灭然而又似乎同时并存;所有这些形象一刻不停地变幻和更新,而它们又都复归于悉达多。他看见一条鱼的形象,一条眼睛已黯然无光的垂死的鱼,正极其痛苦地大张着嘴;他看见一张新生婴儿的脸庞,面色赤红,满是皱纹,正张口欲哭。他看见一张杀人凶犯的脸,看见他持刀刺进一个人的身体;而在同一瞬间,他看到这名罪犯双膝跪地,绳索缠身,头被刽子手一刀砍落。他看到陷于炽烈情爱之狂喜中的男人、女人不同姿态的赤裸身躯;他看到人们死去之后身体僵直、死寂、冰冷而空无。他看到许多动物的形象——野猪、鳄鱼、大像、公牛与飞鸟,他也看到大神黑天 与阿耆尼 。他看到所有这些面宠与形象以千万种方式彼此联系,彼此扶助,彼此爱恋,而同时却又彼此仇恨,彼此毁灭并重获新生。每一形态都终归于无,都是无常世界中苦难而充满激情的存在;然而它们却并未死去,它们只是在不断变幻,不断再生,不断以新的形态出现;只有时间作为不同形态之间联系的纽带。所有这些形态都经历暂住,流转与再生,彼此交汇并融合,而在这一切之上回旋着某种稀薄、虚幻却又无可置疑的存在,像一层薄薄的冰或玻璃体,像一层透明如水的躯壳、外形或面具——而这面具正是侨文达在那一刻所吻的悉达多微笑的面容。侨文达看到这面具般的微笑,这回旋于流转万相之上的圆融的微笑,这超脱于万千生生死死之上的永恒的微笑——悉达多的微笑——与他曾经上百次带着敬畏瞻仰过的乔达摩佛陀的微笑毫无二致。他们的微笑同样是那么安详、微妙而不可测度,同样是那种或许是慈悲,或许是嘲讽,形态万千的圣明的微笑。侨文达知道,一切圆成的佛陀世尊正是如是微笑的。
Recent works have explored aligning images and videos with LLM for a new generation of multi-modal LLMs that equip LLMs with the ability to understand and reason about 2D images.
但是仍缺少对于3D物理空间进行分析的模型, which involves richer concepts such as spatial relationships, affordances, physics and interaction so on.
由此提出了inject the 3D world into large language models
, 介绍一个全新的3D-llm模型族,可以将3D表示(即带有功能的3D点云)作为输入,并执行一系列与3D相关的任务。
优势:
挑战
爱心屋签到: aixinwu.sjtu.edu.cn/products/asw-store
每日二GRISSO💊
Starting from 2/20:
Time | Monday | Tuesday | Wednesday | Thursday | Friday | Saturday | Sunday |
---|---|---|---|---|---|---|---|
08:00 | |||||||
09:00 | |||||||
10:00 | Upgrade linux | 计算机视觉DSY115 | |||||
11:00 | 组会 | ||||||
12:00 | |||||||
13:00 | |||||||
14:00 | 自然辩证法DSY202 | ||||||
15:00 | |||||||
16:00 | |||||||
17:00 | |||||||
18:00 | 数据挖掘CRQ219 | ||||||
19:00 | |||||||
20:00 | |||||||
21:00 | |||||||
22:00 | |||||||
Credits: 3+3+1 |
Moved to [[2025 Summer Schedule]]
Moved to [[2025 Summer Schedule]]
Moved to [[2025 Summer Schedule]]
Momentum Contrast for Unsupervised Visual Representation Learning
伪代码:
1 | # f_q, f_k: encoder networks for query and key |
在使用key encoder(momentum encoder)创建负样本,并把encode过的负样本存在一个queue(FIFO)中方便后续对比时直接使用,每次训练都会使用一个新的mini batch,此时会将此mini batch中的样本encode之后加入queue并删除存在最久的那个mini batch的样本(因为考虑到最老的mini batch使用的encoder是最过时的,所以FIFO是非常合理的),这样可以有效控制负样本的数量,也就是公式中的K。
因为负样本数量(字典/队列)很大,所以没办法给key encoder回传梯度,所以可以考虑把query encoder的参数直接复制给key encoder,但过快改变的key encoder会导致样本字典的特征不一致,所以使用动量更新的方式。
> queue这个字典越大,那么理论上这个m就需要越大,保证字典中key的一致性b)
只有一个编码器进行学习。Memory bank存下了所有样本的key。每当梯度回传后,会把memory bank被本次训练中被采样过的key使用新的encoder进行更新。
MoCo和memory bank 更接近,但是使用了queue dictionary和momentum update