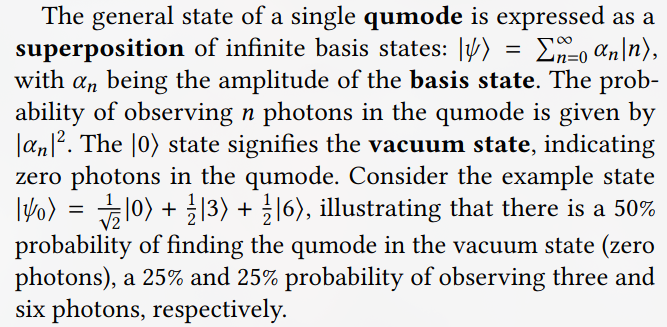
ProxiML -- Building Machine Learning Classifiers for Photonic Quantum Computing
https://dl.acm.org/doi/pdf/10.1145/3620666.3651367
Background
Qumode
Qumodes are a different way of carrying and manipulating quantum information than qubits.
就像二进制在电脑中的encoding方式,总共n bit的内存,那必然只能有 $2^n$ 种内存state,这是由二进制0或1的特性决定的。然后在让我们看qubit和qumode
States for qubits
If we go to qubits, not much in this picture changes. While a qubit has infinitely many possible states, it turns out that you should look at what is called the basis of the state space, which loosely said means that you should find the minimal number of states in which you can express every other state. For a qubit, this turns out to be two, for example the up state and the down state. To use the language from above, each qubit therefore has 2 ‘possible assignments’, and you have n of them, so by the arguments presented above, there are $2^ⁿ$ unique states. Because we are doing quantum mechanics, superpositions of these states are also allowed, but that doesn’t change the picture: the dimensionality of the system is still $2^ⁿ$.
Qubits是由单个光子的量子态决定的,的存储维数限制依然是 $2^n$