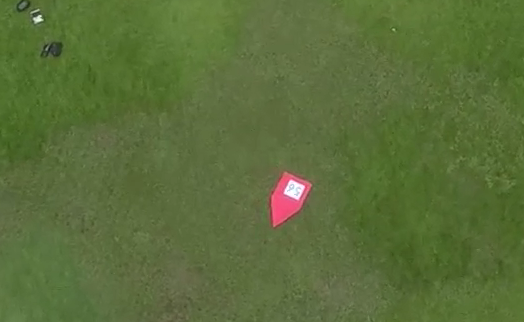
Beautiful Soup
Generate bs4
1 | soup = bs4.BeautifulSoup(text) |
find node
1 | soup.find("div", attrs={"id":"..."}) |
siblings
1 | soup.find("...").next_sibling |
with Regular Expression
1 | soup(class_=re.compile('item-')) |
1 | soup = bs4.BeautifulSoup(text) |
1 | soup.find("div", attrs={"id":"..."}) |
1 | soup.find("...").next_sibling |
1 | soup(class_=re.compile('item-')) |
1 | re.sub(pattern,repl,str) |
1 | df['column_name'].str.contains('pattern') |
Extracting room numbers from a ‘Description’ column in a DataFrame using regular expressions:
Import the re
module:
1 | import re |
Use the apply()
method to apply the function to the ‘Description’ column and create a new ‘RoomNumber’ column:
1 | df['RoomNumber'] = df['Description'].apply(extract_room_number) |
This will extract the room number from the ‘Description’ column and store it in the new ‘RoomNumber’ column.
File Path: catkin_ws/src/test/script/env_pkl_generator.py
1 | cd ~ |
It will open Gazebo and RViz and display the robotic arm on both sofware.
1 | cd ~ |
向日葵
识别吗
341 866 266
ge6v9Q
magma-nvim
Magma is a NeoVim plugin for running code interactively with Jupyter.
Jupynium.nvim
It’s just like a markdown live preview, but it’s Jupyter Notebook live preview!
Jupynium uses Selenium to automate Jupyter Notebook, synchronising everything you type on Neovim.
Never leave Neovim. Switch tabs on the browser as you switch files on Neovim.
CADC Ground Investigation Visual Solution
We want to apply computer vision to the plane to fullfill ground investigation task automatically.
The input image looks like this:
And we hope the output prediction to be 56.
(requirment.txt will be added afterwards)
Basic OpenCV methods is required.
Recommended learning site:
Source code
in detect.py
, there are two large function
1 | def detect_target(image): |
detect_target
receive image data(three channels, BGR), detect the colored targets and returns the index of them.
index:
rect
, indicating the information of the minimum bounding rectangleNote: What is Box2D type rect
?
index | description |
---|---|
rect[0] | the location of the box’s center (x,y) |
rect[1][0] | width of the box(the length of the side which will be first reached by the horizontal line when rotating counter-clockwise) |
rect[1][1] | height |
rect[2] | rotation angle |
First we need to convert the color layout from BGR to HSV, which is easier to judge color.
1 | image_hsv=cv2.cvtColor(image_cut,cv2.COLOR_BGR2HSV) |
Then define a mask that filter the color(hue) range from 160-179 or 0-10 (red),
1 | red1=np.array([0,100,100]) |
And apply the mask to the picture
1 | after_mask=cv2.add(image_cut, np.zeros(np.shape(image_cut), dtype=np.uint8), mask=mask) |
Turn the after mask image into binary image and adapt close and open processing to fill holes and cancel noise.
1 | kernel=np.ones([3,3],np.uint8) |
Then, find the contours of the processed image.
For each contour, adapt filters such as area, the ratio of the height and width of bounding rectangle.
If these restrictions are fullfilled, append the index of bounding rectangle and min area rectangle to two lists respectively, and return the lists.
1 | contours, hier=cv2.findContours(Open,cv2.RETR_EXTERNAL,cv2.CHAIN_APPROX_NONE) |