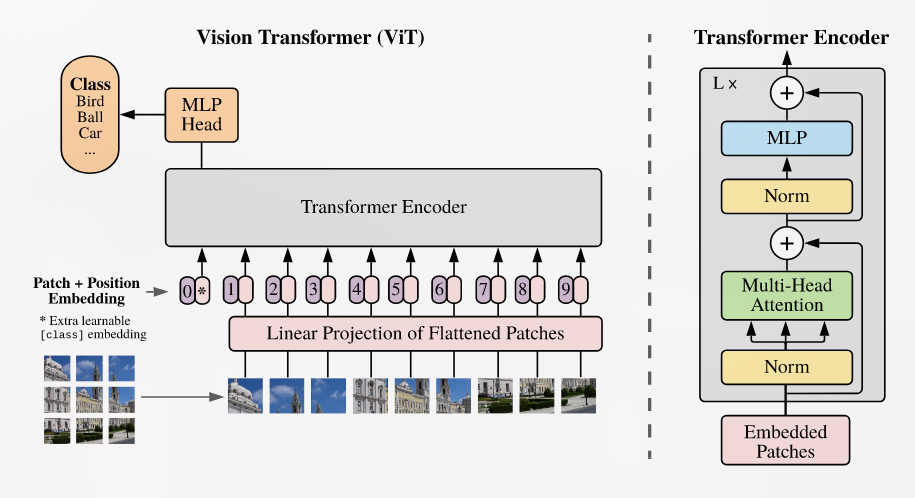
Vision Transformers Need Registers
AN IMAGE IS WORTH 16X16 WORDS- TRANSFORMERS FOR IMAGE RECOGNITION AT SCALE
https://www.youtube.com/watch?v=j3VNqtJUoz0&t=16s
核心思想:
https://github.com/facebookresearch/dino/tree/main
# Emerging Properties in Self-Supervised Vision Transformershttps://juejin.cn/post/7224738994825789496
https://www.youtube.com/watch?v=h3ij3F3cPIk&t=1005s
DI+NO(蒸馏+No Label)
具体来说,DINO 是使用一种称为“无监督自蒸馏”的方法,该方法通过自监督学习来学习模型的知识表示。在这个方法中,模型使用自身的输出来生成“伪标签”,然后使用这些伪标签来重新训练模型,从而进一步提高模型的性能和泛化能力。
https://blog.csdn.net/xbinworld/article/details/83063726
重点idea就是提出用soft target来辅助hard target一起训练,而soft target来自于大模型的预测输出。这里有人会问,明明true label(hard target)是完全正确的,为什么还要soft target呢?
hard target 包含的信息量(信息熵)很低,soft target包含的信息量大,拥有不同类之间关系的信息(比如同时分类驴和马的时候,尽管某张图片是马,但是soft target就不会像hard target 那样只有马的index处的值为1,其余为0,而是在驴的部分也会有概率。)[5]
这样的好处是,这个图像可能更像驴,而不会去像汽车或者狗之类的,而这样的soft信息存在于概率中,以及label之间的高低相似性都存在于soft target中。但是如果soft targe是像这样的信息[0.98 0.01 0.01],就意义不大了,所以需要在softmax中增加温度参数T(这个设置在最终训练完之后的推理中是不需要的)
关于DINO中发生的涌现
https://juejin.cn/post/7280436457142501388
DINO之前的工作
We have also seen emerged two properties that can be leveraged in future applications: the quality of the features in k-NN classification has a potential for image retrieval. The presence of information about the scene layout in the features can also benefit weakly supervised image segmentation.
https://blog.csdn.net/h661975/article/details/135116957
loss: ITC (Image Text Contrastive)
1 | # image_encoder - ResNet or Vision Transformer |
Cross_entropy_loss:
CLIP 本质上是全局图像嵌入,不利于像素对齐特征提取。
LERF- Language Embedded Radiance Fields
NeRF+CLIP
一个Language Field
通过优化从现成的视觉语言模型(如 CLIP)到 3D 场景的嵌入,为 NeRF 中的语言奠定基础。
LERF 提供了一个额外的好处:由于我们从多个尺度的多个视图中提取 CLIP 嵌入,因此通过 3D CLIP 嵌入获得的文本查询的相关性图与通过 2D CLIP 嵌入获得的文本查询的相关性图相比更加本地化。根据定义,它们也是 3D 一致的,可以直接在 3D 字段中进行查询,而无需渲染到多个视图。
相较于Clip-Field[[CLIP-Fields- Weakly Supervised Semantic Fields for Robotic Memory]], LERF 更密集。
CLIP-Fields [32] and NLMaps-SayCan [8] fuse CLIP embeddings of crops into pointclouds, using a contrastively supervised field and classical pointcloud fusion respectively. In CLIP-Fields, the crop locations are guided by Detic [40]. On the other hand, NLMaps-SayCan relies on region proposal networks. These maps are sparser than LERF as they primarily query CLIP on detected objects rather than densely throughout views of the scene. Concurrent work ConceptFusion [19] fuses CLIP features more densely in RGBD pointclouds, using Mask2Former [9] to predict regions of interest, meaning it can lose objects which are out of distribution to Mask2Former’s training set. In contrast, LERF does not use region or mask proposals.
Some Thoughts Regarding -Reconstruct Anything-
主要记录一些读场景语义化重建的论文的过程中的想法
限定:暂不考虑机器人的移动性,也就是不需要跨视野的导航(OK-Robot),暂定为桌面机器人
具体来说,通用机器人的特点包括:
受DINO自蒸馏自监督的启发,可以通过物体活动的图像序列来推测物体各个部分的物理关系(attention map)[[DINO]]
训练集可以使用Unity生成不同的光影/物体,连接语义
voxel collider for detected objects, joints, physics agent interact with physics engine.
点云数据,grounded caption=>object property, hierarchy relation, joints(maybe new model should be proposed)
受[[BLIP]]启发,understanding for language & existing point cloud, generation for the rest of the point cloud (Wonder3D已实现)
CLIP-Fields- Weakly Supervised Semantic Fields for Robotic Memory
疑问:
A spatial-semantic memory
是一个隐式场景模型,可用于各种任务,例如分割、实例识别、空间语义搜索和视图定位
CLIP-Fields 学习从空间位置到语义嵌入向量的映射。
这种映射可以仅通过来自网络图像和网络文本训练模型(例如 CLIP[[CLIP多模态预训练模型]]、Detic 和 Sentence-BERT)的监督进行训练;因此不使用直接的人类监督。
We aim to build a system that can connect points of a 3D scene with their visual and semantic meaning.
Provide an interface with a pair of scene-dependent implicit functions $f, h : R^3 → R^n$ such that for the coordinates of any point P in our scene, f (P ) is a vector representing its semantic features, and h(P ) is another vector representing its visual features.
貌似每针对一个新场景都需要重新train一遍来获得坐标到语义的映射。
OK-Robot- What Really Matters in Integrating Open-Knowledge Models for Robotics
Creating a general-purpose robot has been a longstanding dream of the robotics community.
当前想要实现这一目标的系统脆弱、封闭,并且在遇到未见过的情况时会失败。即使是最大的机器人模型通常也只能部署在以前见过的环境中 [5, 6]。在机器人数据很少的环境中,例如在非结构化的家庭环境中,这些系统的脆弱性会进一步加剧。
虽然大型视觉模型显示出语义理解 、检测以及将视觉表示与语言联系起来的能力并且与此同时,机器人的导航、抓取和重新排列等基本机器人技能已经相当成熟。
但是将现代视觉模型与机器人特定基元相结合的机器人系统表现非常差。
这可能是因为单纯将多个不确定性的系统组合在一起会导致准确率急剧恶化。
所以我们需要一个将VLM和机器人primitives(导航,抓取,放置)结合在一起的细致框架,即OK-Robot。
Pick up A (from B) and drop it on/in C”, where A is an object and B and C are places in a real-world environment such as homes
负责空间重建,识别物体大致位置,机器人导航
用到的方法: